Zoom on AI for line inspection
Published on 10 February 2025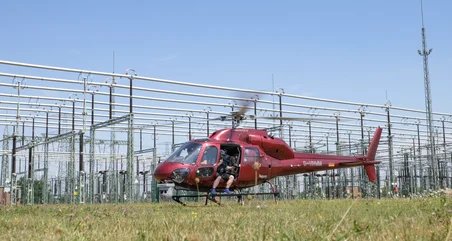
.jpga38d.jpg)
Explore the Artificial Intelligence for Line Inspection (AILI) project, a pioneering initiative that uses AI to improve the efficiency and accuracy of overhead power line inspections, through the interview of Thorsten WERNER, Head of Business Development in the CityNetworks & Grids division at SPIE Germany Switzerland Austria.
The Artificial Intelligence for Line Inspection (AILI) project within SPIE involved developing AI-supported image recognition software to automatically identify damaged components on overhead power lines when inspections are performed from helicopter.
Analysis is supported by a dashboard that consolidates data from various sources and formats (photos, videos, position metadata, laser scans, etc.) and displays the identified components, as well as damage or other anomalies. Relevant experts can then assess the severity of the damage, define possible corrective measures, and share tailored information that becomes integrated to customers’ asset management system.
In your view, how has AILI impacted your daily routine or workflow?
Before, our workflow involved a lot of tedious data gathering from various sources and manual, unstructured analysis of photos and videos. The difficulty in identifying components and objects from a mass of data led to increased manual effort and error rates. Now, we have clear, automated processes that efficiently and accurately distinguish between machine learning and human inputs.
Would you say AILI improves collaboration and communication with internal and external stakeholders?
Certainly. It became very apparent during meetings with customers: we’ve seen very positive reactions and a lot of interest on their part. AILI opens completely new opportunities for them: we are after all talking about a project that requires covering approximately 500,000 components and/or parts on overhead power lines! Manually, sending people around with notepads to record defects from the naked eye would be a huge undertaking. There’s also the safety risk of climbing power masts, which we can avoid completely by taking photos from helicopters. Merging and bundling hand-gathered data into one structure would be another challenge. All in all, the whole thing would take months and some information wouldn’t be available at all.
How about the solution’s operational impact? Can you share any benefits in terms of productivity, resource optimisation, and the like?
Internally, we’ve seen a 30% efficiency increase; we’re also operating at a much higher degree of accuracy, which in turn improves quality, of course. Meanwhile, we’ve reduced helicopter rental costs by €2,000/h and emissions by 300 kgCO2e/h.
Use case
AILI supports the development of flexible grids, i.e. capable of adapting power transmission capacity to the prevailing weather conditions. Flexible and reliable power transmission grids are key to meeting the challenges of energy transition.
Benefits
- Automated, accurate fault detection
- More effective & faster inspections backed by harmonised data
- Automated component recognition
- Geolocation of defects & generation of structured reports to support maintenance teams
- Thousands of tons of carbon saved
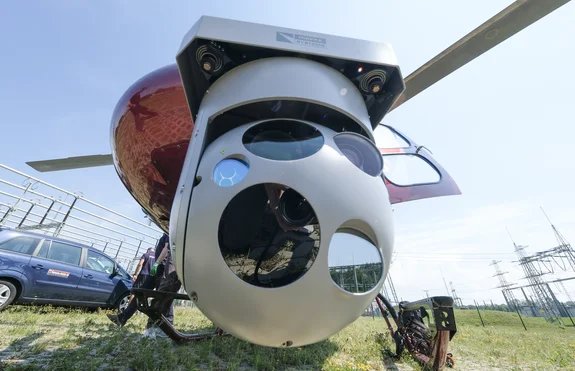
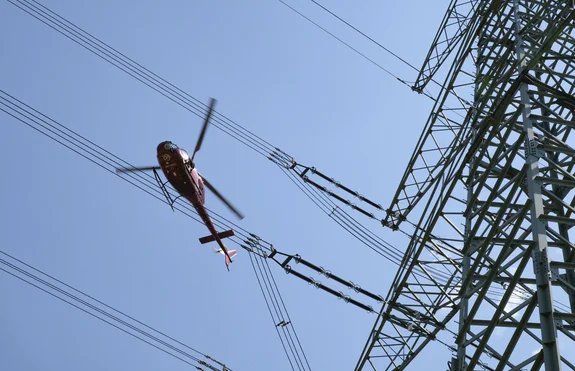
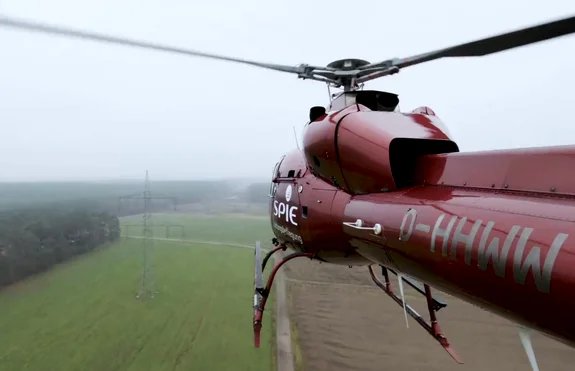
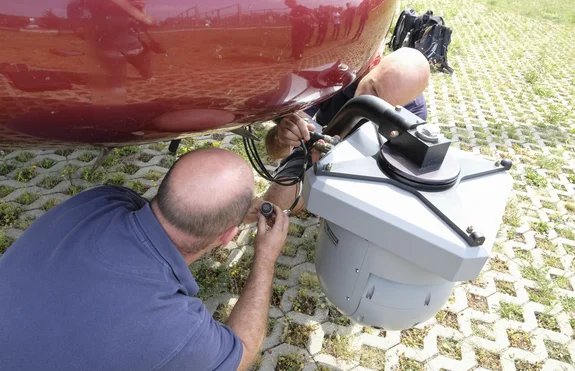
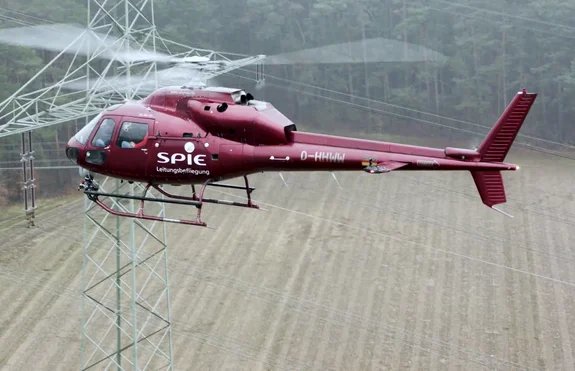
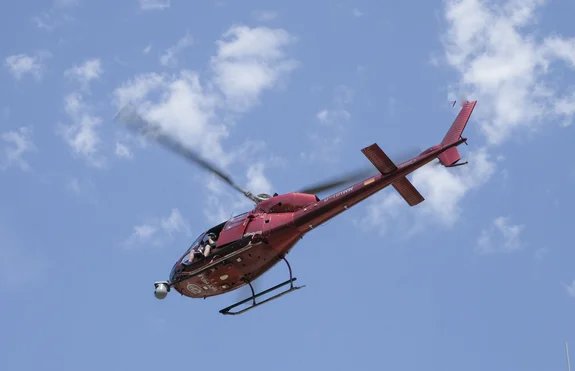
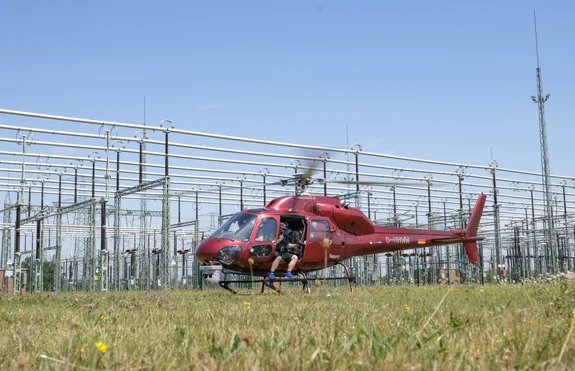